Understanding the Concept of Machine Learning Data Labeling
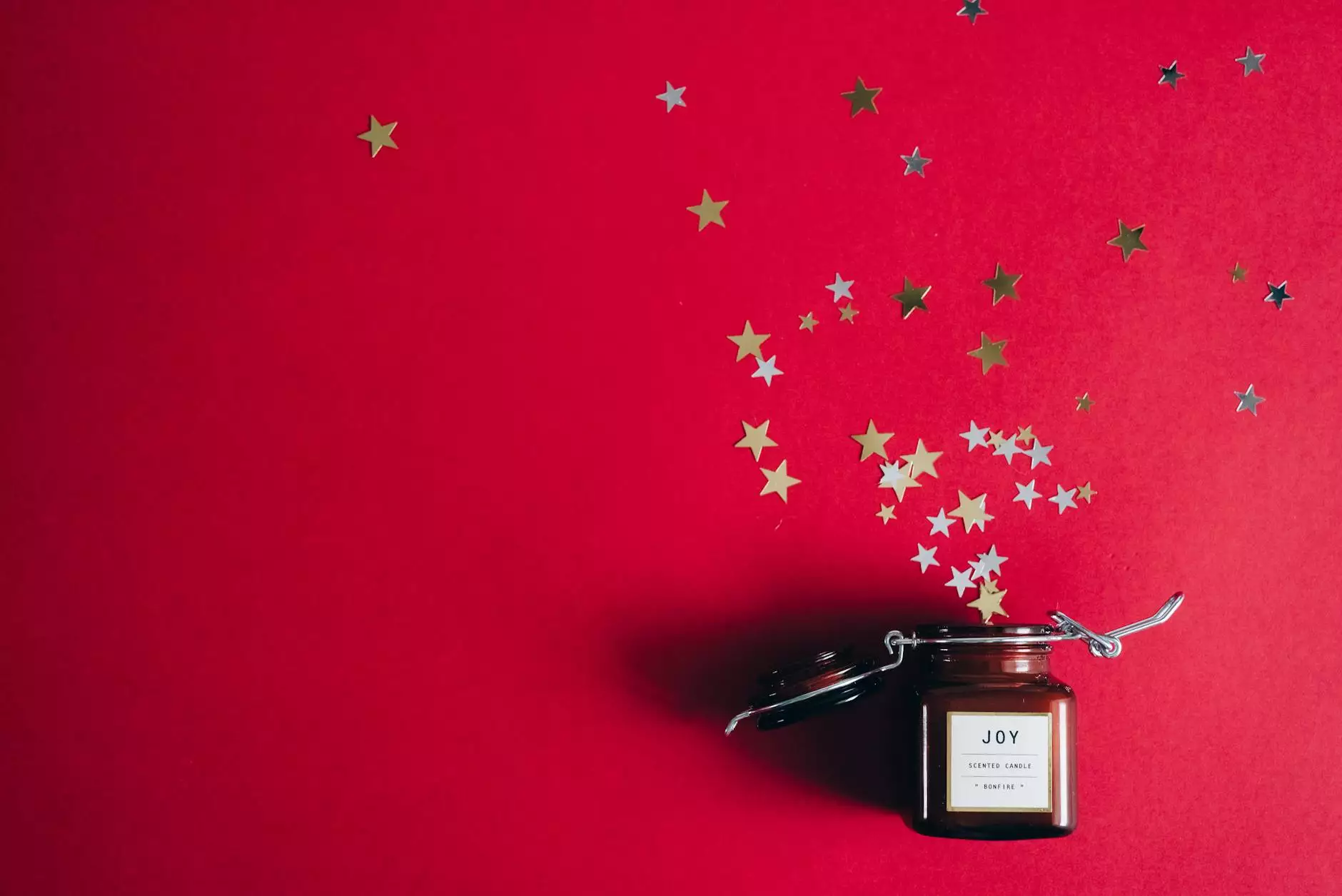
Machine learning data labeling is an essential process that dictates the success and accuracy of machine learning models. As artificial intelligence (AI) gains momentum across various industries, the necessity for precise and high-quality data annotation is more critical than ever.
What is Data Labeling?
Data labeling is the process of assigning tags or labels to data samples so that machines can recognize them. This crucial step allows models to learn from trained data and makes it easier to identify patterns when processing new, unlabeled data.
Why is Machine Learning Data Labeling Important?
The importance of machine learning data labeling in business can be summarized as follows:
- Model Accuracy: Proper labeling ensures that the machine learning model can correctly interpret and classify the data, resulting in higher accuracy and performance.
- Training Efficiency: Quality labels help streamline the training process, reducing the time taken to achieve optimal model performance.
- Data Diversity: A diverse set of labeled data provides a robust foundation for generalizing models to perform well across various scenarios.
- Real-World Applications: With accurate labels, machine learning can be applied effectively to real-world problems, including healthcare, finance, and autonomous vehicles.
Types of Data That Need Labeling
Different types of data require different labeling techniques. Some common examples include:
- Text Data: Used in natural language processing (NLP), this includes sentiment analysis, translation, and information extraction.
- Image Data: Useful for computer vision tasks such as object detection, facial recognition, and image segmentation.
- Audio Data: Applied in speech recognition and voice analysis.
- Video Data: Essential for action recognition and scene understanding.
Challenges in Machine Learning Data Labeling
Machine learning data labeling comes with several challenges that businesses must address to ensure quality results:
- Consistency: Maintaining consistency in labeling across large datasets can be difficult, especially when multiple annotators are involved.
- Quality Control: Ensuring the accuracy of labels requires rigorous quality assurance processes.
- Scalability: As datasets grow, scaling the labeling process without compromising quality becomes a daunting task.
- Cost: High-quality data labeling involves significant resources, both in terms of time and financial investment.
Solutions for Effective Data Annotation
To tackle the challenges associated with machine learning data labeling, businesses can adopt various strategies:
- Utilizing Data Annotation Tools: Advanced data annotation tools, like those offered by keylabs.ai, streamline the labeling process and ensure better quality results.
- Employing a Skilled Workforce: Using experienced annotators proficient in the specific data domain leads to better quality labels.
- Implementing Quality Assurance Processes: Establishing a rigorous QA framework that includes peer reviews, audits, and feedback loops enhances overall labeling accuracy.
- Leveraging Automation: Where possible, incorporating automated labeling solutions can greatly accelerate the process.
The Role of KeyLabs.ai in Data Annotation
KeyLabs.ai specializes in providing top-notch data annotation tools and platforms that allow businesses to effectively manage their machine learning data labeling needs. Here’s how our solutions can transform your data annotation:
1. Comprehensive Data Annotation Tools
Our data annotation tools are designed to cater to various data types including text, images, audio, and video. These tools offer intuitive interfaces that simplify the labeling process, making it accessible even to those with minimal technical expertise.
2. Customizable Annotation Solutions
Every business has unique needs. At KeyLabs.ai, we provide customized annotation solutions that can be tailored to fit your specific project requirements. This flexibility ensures that you receive precisely the data you need, labeled correctly for optimal model training.
3. Quality Assurance Mechanisms
We employ robust quality assurance mechanisms to ensure that the data labeling process meets industry standards. Our system includes multiple rounds of review, allowing for errors to be caught and corrected before they impact your machine learning models.
4. Scalable Solutions
As your data grows, so do our capabilities. Our platforms are designed to scale with your needs, facilitating efficient labeling for large datasets without sacrificing quality. This scalability is vital for businesses experiencing rapid growth or changing data needs.
5. Commitment to Data Privacy
We understand the importance of data security and compliance. KeyLabs.ai takes data privacy seriously, implementing strict protocols to protect your sensitive information throughout the data labeling process.
Conclusion: The Future of Machine Learning Data Labeling
In conclusion, machine learning data labeling is a foundational aspect of developing efficient and accurate machine learning models. With the rise of AI and continual improvements in technology, the demand for quality data annotation will only increase. By partnering with experienced providers like KeyLabs.ai, businesses can effectively overcome the challenges of data labeling, enjoy streamlined processes, and achieve superior results in their machine learning initiatives.
Call to Action
Are you ready to elevate your data annotation processes? Visit KeyLabs.ai today to explore our comprehensive suite of data annotation tools and platforms designed to meet your business needs!